Because of its usefulness, AI is now a key component in all industries, and AI annotator are currently assisting with AI model training. AI annotation is labeling data—image, audio, text, or video—to enable machine learning algorithms to detect patterns and subsequently optimize their precision.
After reading this guide, AI annotator can jumpstart their careers. It includes the tools, skills, and best practices crucial for success.
Who is an AI Annotator?
Data that AI Annotators bedeck become the gears in a well-functioning machine, designed to help AI Models run as efficiently within an enterprise context. They are fundamental pieces for many companies, and they range from designing the frameworks of annotation tools to collecting, cleaning, and labeling data that will teach AI models how to perform various enterprise tasks. The information includes:
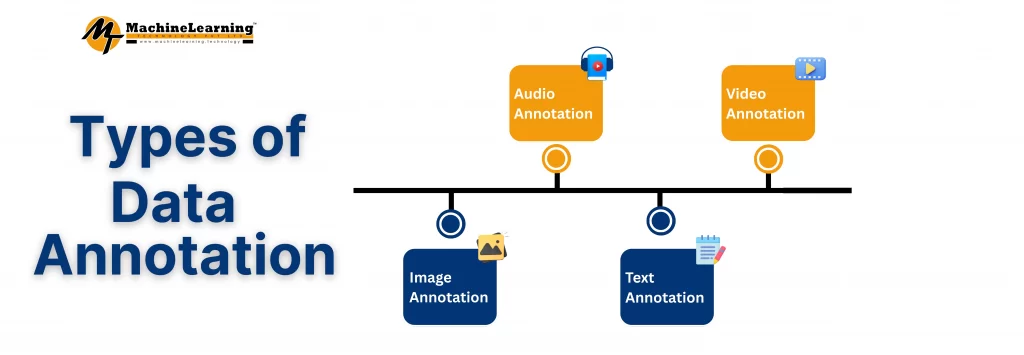
- Text: Categorizing emails as spam or non-spam.
- Images: Identifying objects in photos (e.g., cars, humans, animals).
- Audio: Transcribing speech into text.
- Video: Tracking objects in motion (e.g., pedestrian detection for autonomous vehicles).
Why is AI Annotation Important?
AI Annotation is critical for multiple reasons, especially when it comes to constructing and making improvements to AI models. Here are ways in which thinking AI systems are essential:
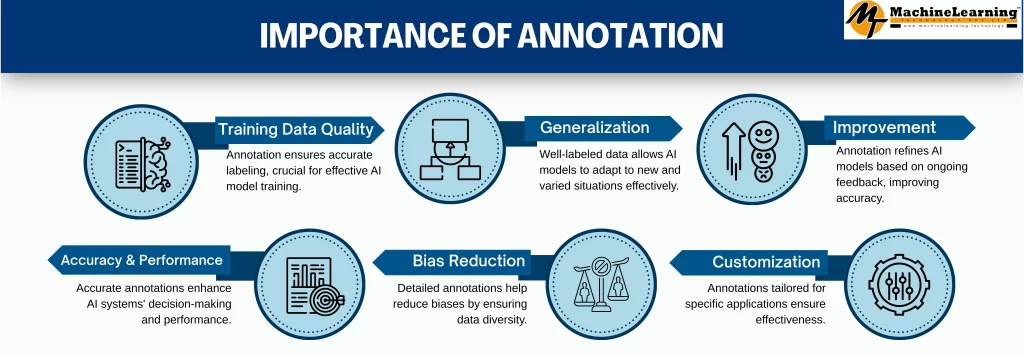
- Training Data Quality: Annotation involves labels within the data that AI models learn from. These labels include image tags, audio files, and even written texts. To efficiently train models, authentic and accurate annotations are fundamental.
- Model Accuracy and Performance: The AI’s results must maintain model accuracy. AI systems’ performance depends upon the labels and data provided to them. Properly labeled data dramatically increases the set’s decision-making ability when supplied with new data.
- Model Generalization: AI models adapt well to newly encountered situations due to well-labeled data that covers plenty of scenarios, variations, and exceptions. This is very important for the strength of AI systems in real-life applications.
- Bias Reduction: By paying attention to detail in annotation and ensuring the data covers different demographics and situations, we can avoid bias in AI models. This aids in building less biased AI systems.
- Continuous Improvement: Annotation serves as a tool during the refinement and upkeep of the model. Based on feedback from model outputs, new annotations can be created, which can subsequently be used to retrain and enhance the model.
- Specificity and Customization: AI systems must use distinct licenses, permissions, specialized annotations, and target files when focusing on specific markets or tasks, such as medical diagnosis and autonomous driving, to ensure effective deployment.
Skills Required to Become an AI Annotator
An AI annotator is a blend of a techie and a non-techie. These are the standalone skills that are typically required:
- Attention to Detail: This is critically important as the effectiveness of the AI model is highly dependent on annotations. Annotators must pay attention to smaller details of the data they are working with, such as distinguishing between subtle features within images or text.
- Patience and Diligence: Annotation is often monotonous and time-consuming. The ability to concentrate for long periods is essential.
- Essential Technical Skills: Certain tasks may involve technical skills, so possessing some technical skills will help. Some experience with computers and standard computer programs is usually a requirement.
- Understanding of Data: Annotators have to appreciate the data features that may pose some challenges, particularly for specialized fields with domain knowledge, such as surgically implanted imaging or legal files.
- Problem-Solving Skills: It is helpful to understand the issues that stem from the annotation process and know how to tackle them, especially when ambiguity clouds judgment.
- Communication Skills: Annotators must interact and provide feedback to the other team members, such as comments regarding the annotation procedures or clarifications about the rules.
- Adaptability: As the project’s requirements change, the tools and boundaries within which the work is completed will also change. It is crucial to be able to work with new software, other methods, or even data types.
- Domain-Specific Knowledge: Certain fields, such as medicine and healthcare, autonomous cars, and legal frameworks of Artificial Intelligence, have very specific requirements and/or knowledge skills that aim to ensure the annotations created are correct and useful.
Tools Used in AI Annotation
Based on the type of data you have, multiple AI annotation tools are available to assist you. Here are some of the most important ones:
Text Annotation Tools
- Prodigy: AI-powered text annotation for NLP tasks.
- LightTag: Collaborative writing and text labeling tool.
- Labelbox: Customizable annotation templates.
Image Annotation Tools
- LabelImg: Open-source image annotation tool for object detection.
- Supervised: Online application for video and image annotation.
- VGG Image Annotator (VIA): A free tool for image annotation.
Audio Annotation Tools
- Audacity: Edit and annotate audio files using this free tool.
- Praat: Annotation and analysis of phonetics are predominantly done using this software.
- Sonix AI: The annotation of the audio-to-text conversion is done automatically.
Video Annotation Tools
- CVAT: This open-source tool is designed for video labeling.
- V7 Labs: Video annotation platform that uses AI.
- Makesense.ai: Provides free segmentation and bounding box annotation tool.
Suggested Approaches for AI Annotation
Adhere to the following suggestions to guarantee quality data annotation:
Follow Annotation Guidelines
- Familiarize yourself with the annotation policies before beginning work.
- Follow the rules of uniformity for precise labeling.
Use High-Quality Data
- Handle organized and clean datasets.
- Avoid mistakes like missing labels and misclassification.
Maintain Consistency
- Uniform labeling throughout datasets aids AI learning.
- Use the known tags and categories offered.
Collaborate with Teams
- When working in a team set, ensure everyone is clear on the level of standardization required.
- Take advantage of collaboration options in annotation tools.
Regularly Review and Validate Annotations
- Verify that the labels that have already been placed reflect the annotation appropriately.
- Peer review to improve data accuracy.
Automate When Possible
- Tools with built-in AI automation are available.
- Tools powered by AI should be utilized for tedious duties.
Issues Involved in Data Annotation of AI
- Quality and Accuracy: Straightforward accuracy is essential when creating data annotations. Precision is crucial, as AI models depend heavily on it.
- Scalability: Quality should never be compromised while managing the increased volume of annotation data needed.
- Bias and Representation: Inherited biases should not pass through AI models. Diverse datasets should be created without any form of imbalance.
- Cost and Efficiency: Maintaining operational efficiency while balancing the cost of annotation systems is challenging.
- Tool Limitations: Exceeding the functionality and versatility of preset boundaries of the current annotation tools.
- Privacy and Security: Ensure that sensitive data is handled ethically and is secured, especially in areas such as healthcare.
The Future of AI Data Annotation
Concerning the future of AI data annotation, it will aim to automate assistance processes further to minimize the manual effort necessary for the workload. New advanced tools and interfaces are anticipated to be created with better usability and more overt integration. Quality control is expected to advance through automated and AI-aided data accuracy checks. More focus on ethical AI will provide more representative and diverse datasets, whereas the scope of professional development and training will provide a frame for encoders to adopt complex skills. Community-based approaches to AI data annotation will be achieved through collaborative annotation platforms, increasing efficiency and knowledge sharing. The continuous change will profoundly result in the application and effectiveness of AI in different fields, making data annotation a constantly changing and highly relevant area of expertise.
How to Get Started as an AI Annotator
If you are looking to jumpstart your career in AI annotation, this guide is for you:
Learn the Basics
- Fathom the concepts of AI and machine learning.
- Enroll in free online courses specific to data annotation.
Familiarize Yourself with Free Annotation Software
- Test LabelImg, LightTag, and Audacity for instructional purposes.
- Participate in open-source annotation projects for practice.
Build a Portfolio
- Indicate any previous work done in AI annotation.
- Develop a sample project to illustrate your skill set.
Start Looking for Employment or Freelance Opportunities
- Search for available positions on Upwork, Fiverr, and LinkedIn.
- Submit applications to AI firms looking for annotation specialists.
Continue Developing Your Competences in the Long Term
- Consult available materials about current developments in AI.
- Take your skills to the next level by enrolling in higher-level programs.
Career Opportunities in AI Annotation
AI and machine learning are booming fields that have created and will continue to create jobs as AI annotators and as AI and Data Science practitioners. Here are some of the available positions related to AI annotation:
- Data Annotator: This job consists of marking different types of files, such as pictures, text documents, audio files, and videos, so AI systems can be trained on them. It is essential for many industries, including medicine, self-driving vehicles, and retail.
- Quality Control Specialist: Maintain the reliability of AI models by ensuring the accuracy and Consistency of annotations, performing quality checks, and upholding the required standards.
- AI Research Assistant: Assist data scientists in AI research, managing and annotating datasets, and developing and testing AI models.
- Machine Learning Data Specialist: Supervise the organization of datasets required for machine learning, enhancing their quality and making them scalable and easier to use.
- Project Manager: Coordinate and oversee annotation projects, monitoring timelines and resource allocation to ensure success while fulfilling technological and client goals and other various requirements.
- Annotation Tools Developer: Develops and modifies tools for data annotation, emphasizing ease of use and flexibility to adapt to multiple projects.
Conclusion
The field of AI annotation offers numerous opportunities. Those with a good eye for detail, patience, and some technical skills can easily cultivate a career as an AI annotator.
The best way to start is to focus on building up skills, practicing, and gaining experience in annotation tools. Over time, it is possible to grow into the AI sector and contribute to innovative technologies.
Take the first step on the path of becoming an AI annotator today! Start practicing and dive into the fascinating universe of AI!